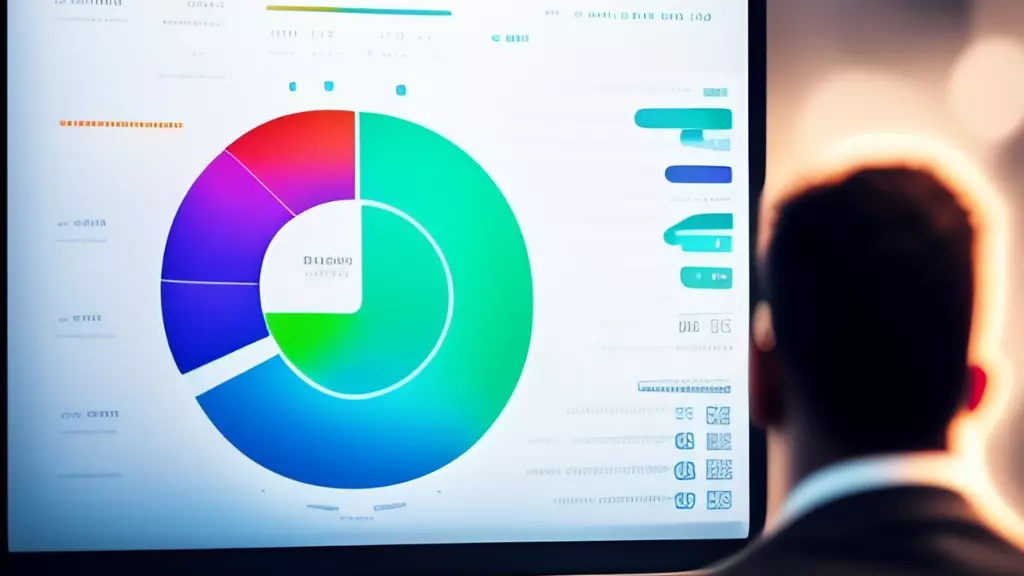
Graphical analytics, also known as graph analytics or network analysis, is an innovative field of study that focuses on analyzing relationships between entities to uncover valuable insights.
Unlike traditional analytics that primarily works with numeric data, graphical analytics delves into the complex web of connections and dependencies among entities, providing a deeper understanding of various systems and processes.
Understanding Graphs: Nodes, Edges, and Relationships
At the core of graphical analytics lies the concept of a graph. Simply put, a graph consists of nodes or vertices, which represent the entities in a system, and edges or links, which represent the relationships between those entities.
This mathematical structure enables the representation and analysis of various types of relationships in physical, biological, social, and information systems.
- Graphs come in different forms, each serving its unique purpose:
- Directed graphs: Also known as digraphs, these graphs depict relationships with directed edges, indicating a one-way connection from one node to another. They are particularly useful for modeling asymmetric relationships.
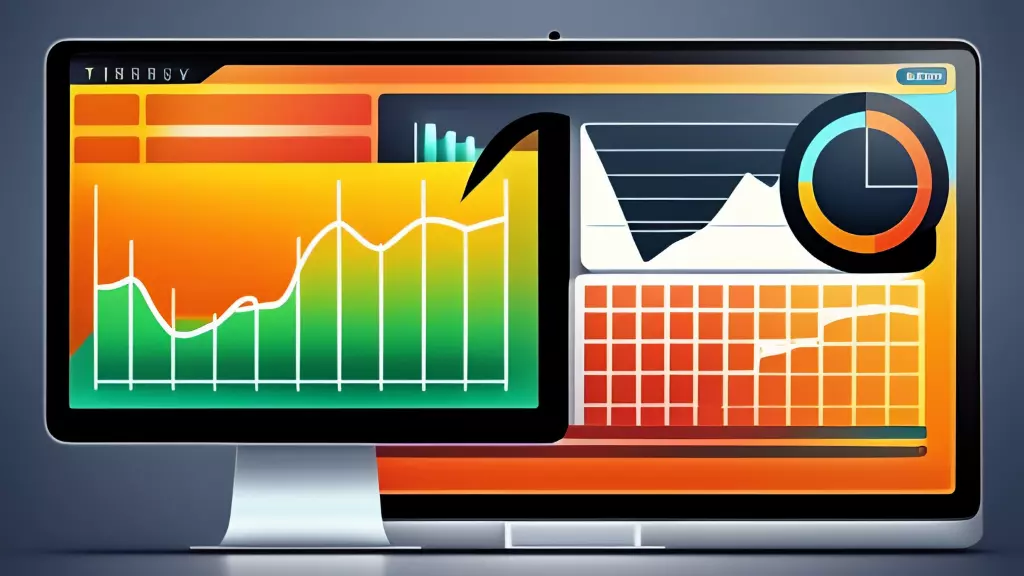
- Undirected graphs: In contrast to directed graphs, undirected graphs represent relationships without indicating a specific direction. They express symmetric relationships between nodes.
- Weighted graphs: Weighted graphs assign numerical weights to edges, providing additional information about the strength or significance of the relationships. These graphs are valuable for solving shortest path problems and other analytical tasks.
- Cyclic graphs: Cyclic graphs contain at least one path that loops back to the starting node. In contrast, acyclic graphs do not contain any cycles. The presence or absence of cycles impacts the analysis and interpretation of the graph.
The Rise of Graphical Analytics
Graphical analytics has gained significant traction across various industries, with organizations recognizing its potential to uncover valuable insights. As businesses grapple with increasing volumes of interconnected data, graph analytics offers a powerful approach to navigate and understand complex relationships. The market for graphical analytics is experiencing substantial growth, with a projected market size of approximately $2.5 billion by 2024.
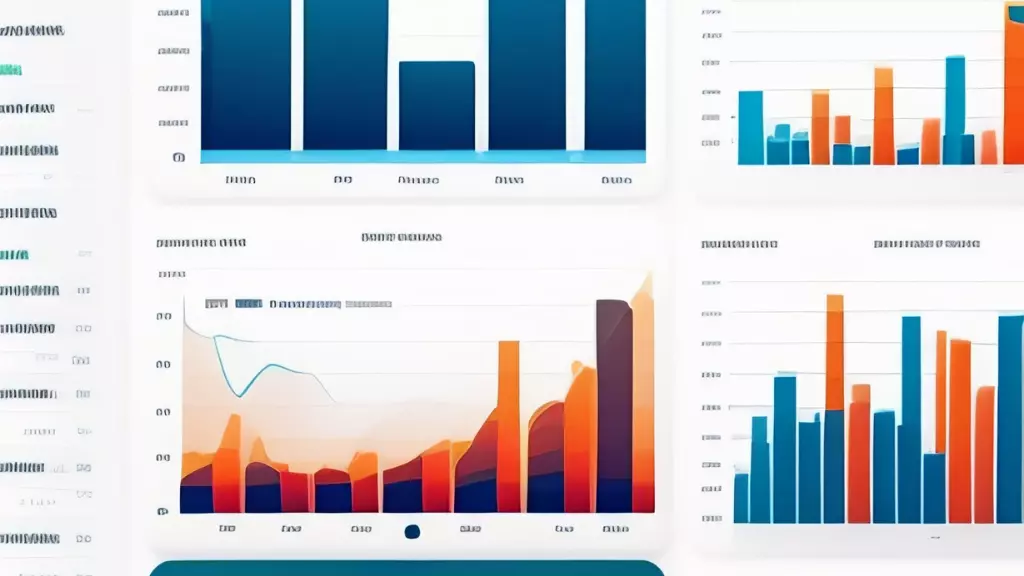
This growth reflects the increasing demand for graph-based insights in fields such as marketing, fraud detection, supply chain optimization, and search engine optimization.
Key Types of Graph Analytics
Graph analytics encompasses a range of techniques and algorithms that enable the analysis of relationships between entities. Each type of graph analytics serves a specific purpose and offers unique insights:
- Centrality Analysis
Centrality analysis estimates the importance of a node within a network based on its connectivity. It helps identify influential individuals or nodes within social networks or frequently accessed web pages through algorithms like PageRank. By understanding centrality, organizations can focus their efforts on engaging with key influencers and optimizing network dynamics.
- Community Detection
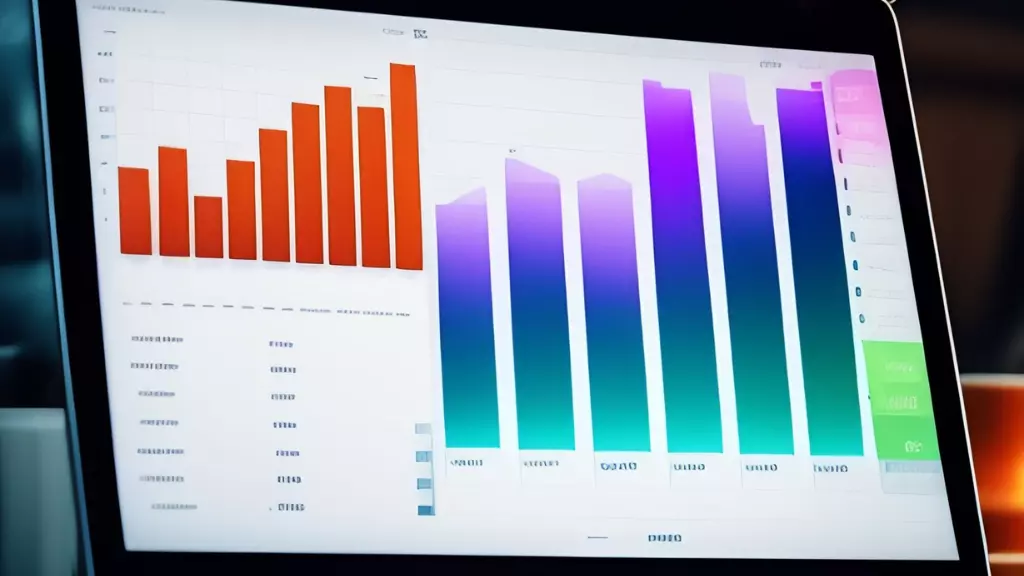
Community detection involves identifying groups of nodes that have dense connections within themselves but sparse connections with other groups. By analyzing the distance and density of relationships, community detection algorithms can uncover clusters or communities within social networks. This insight is invaluable for targeted marketing, understanding group dynamics, and detecting potential fraud or security threats.
- Connectivity Analysis
Connectivity analysis aims to determine the strength or weakness of the connections between two nodes in a graph. It provides insights into the level of influence or dependency between entities. Connectivity analysis is particularly relevant in social network analysis, where understanding the strength of relationships helps identify key influencers or potential collaboration opportunities.
- Path Analysis
Path analysis examines the relationships between nodes in a graph, focusing on finding the shortest or most relevant paths between entities. This type of analysis has applications in transportation logistics, social network analysis, and optimizing various processes. By determining the optimal paths, organizations can enhance efficiency, minimize costs, and improve overall performance.
- Predictive Analysis
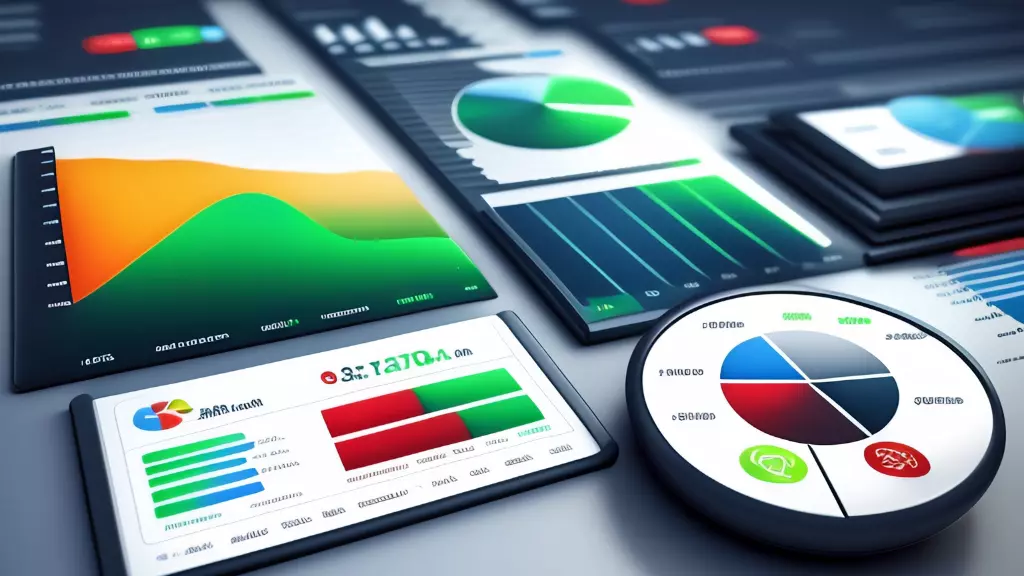
Predictive analysis in graph analytics involves estimating future changes or relationships based on existing graph data. By leveraging proximity and structural patterns within the graph, algorithms can predict new connections or identify hidden relationships. This capability is valuable in fields such as recommendation systems, fraud detection, and supply chain optimization.
Applications of Graphical Analytics
Graphical analytics finds applications in various industries and domains, unlocking insights and driving informed decision-making:
- Journalism
Graphical analytics has revolutionized investigative journalism by enabling the analysis of complex networks of relationships. For example, the International Consortium of Investigative Journalists (ICIJ) utilized graph analytics to uncover the Panama Papers scandal. By analyzing the relationships between offshore companies and their real owners, journalists were able to expose the intricate web of corruption and money laundering.
- Compliance and Fraud Detection
Graphical analytics plays a crucial role in compliance efforts and fraud detection. Government agencies and financial institutions leverage graph analytics to identify potential money laundering activities, trace illicit transactions, and detect connections to sanctioned entities. By analyzing communication data, social media activity, and financial transactions, organizations can uncover non-obvious patterns and mitigate risks.
- Supply Chain Optimization
Optimizing supply chain operations is a complex task, especially considering the numerous entities and dependencies involved. Graphical analytics offers valuable insights into supply chain networks, enabling organizations to identify bottlenecks, optimize routes, and improve overall efficiency. By analyzing the relationships between suppliers, manufacturers, distributors, and customers, businesses can streamline their supply chain operations and enhance customer satisfaction.
- Marketing and Recommendation Systems
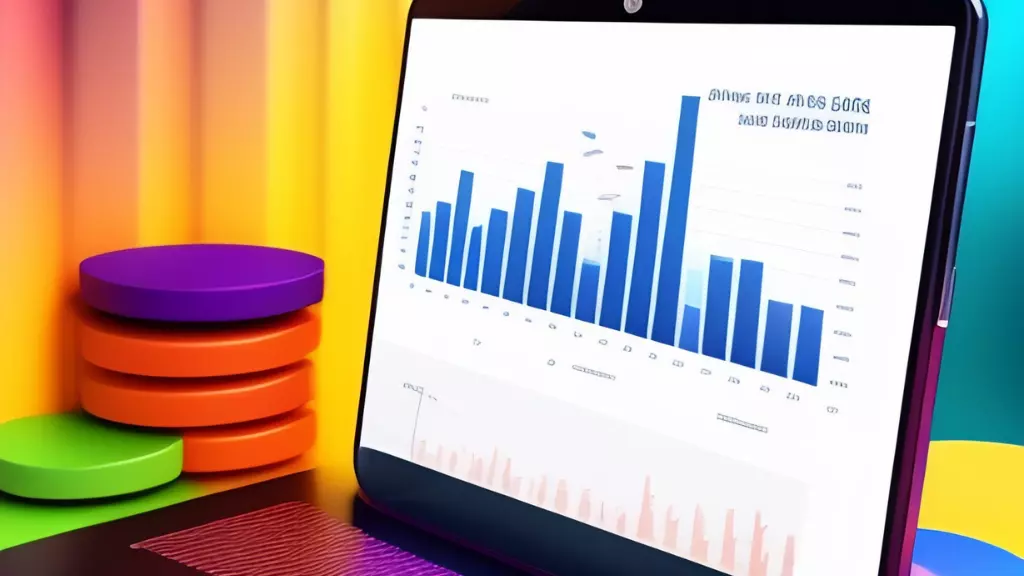
Graphical analytics plays a crucial role in understanding customer behavior, identifying influencers, and optimizing marketing strategies. By analyzing social networks and customer preferences, organizations can build intelligent recommendation systems that deliver personalized recommendations to customers in real-time. This capability enhances customer engagement, drives sales, and fosters brand loyalty.
- National Security
Graphical analytics has found applications in national security, where it is used to detect potential threats and identify unlawful activities. Intelligence agencies analyze communication data and social networks to uncover non-obvious relationships and patterns that may indicate criminal or terrorist activities. By leveraging graphical analytics, law enforcement agencies can enhance their ability to identify and mitigate security risks.
- Healthcare
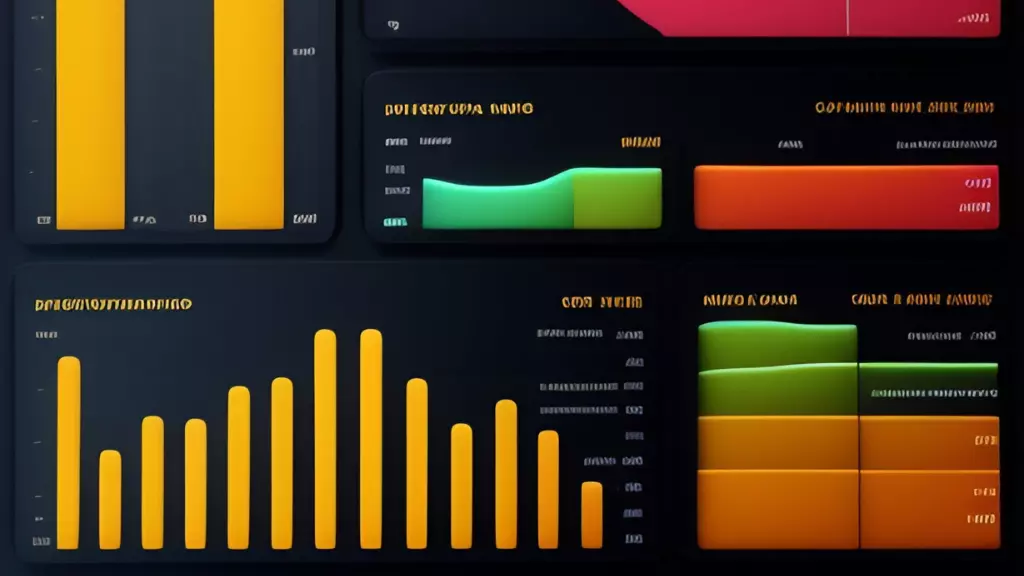
Graphical analytics has proven valuable in healthcare, particularly in pandemic management and containment efforts. Graph databases and analytics tools were employed to track the spread of the virus and identify potential contacts. By mapping the connections between individuals and known carriers, authorities could effectively trace and isolate potential cases, thereby controlling the spread of the virus.
Graphical Analytics: Going Beyond Traditional Analytics
Graphical analytics stands apart from traditional analytics methodologies by focusing on relationships and connections rather than numeric data. While traditional analytics relies on statistics, programming, and operations research, graphical analytics leverages graph-specific algorithms to analyze the complex web of relationships within a graph. To enable the analysis of graph data, graph databases play a vital role. Graph databases provide a flexible and efficient means of storing and querying graph-based data, allowing for seamless exploration and analysis. Leading graph database software tools include ArangoDB, Amazon Neptune, Neo4j, OrientDB, Dgraph, and FlockDB. The future of graphical analytics looks promising, with continued advancements in graph database technology, algorithm development, and the increasing availability of big data.
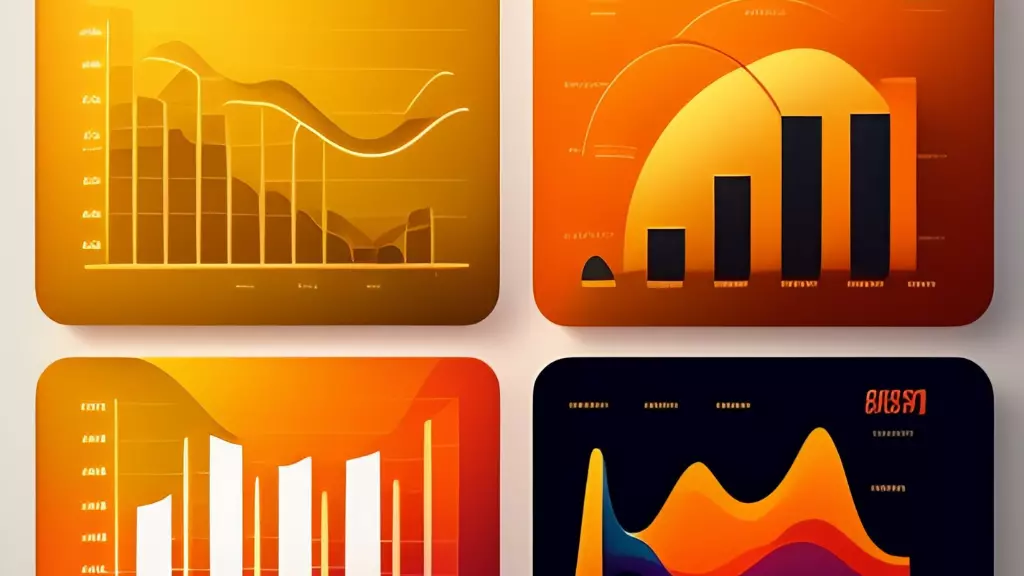
As organizations strive to uncover deeper insights and gain a competitive edge, graphical analytics will undoubtedly play a crucial role in driving informed decision-making and optimizing complex systems.
Conclusion
Graphical analytics offers a powerful approach to understanding and analyzing complex relationships in various domains. By leveraging the insights gained from graphical analytics, organizations can drive innovation, optimize operations, and make informed decisions based on a deeper understanding of their interconnected systems.
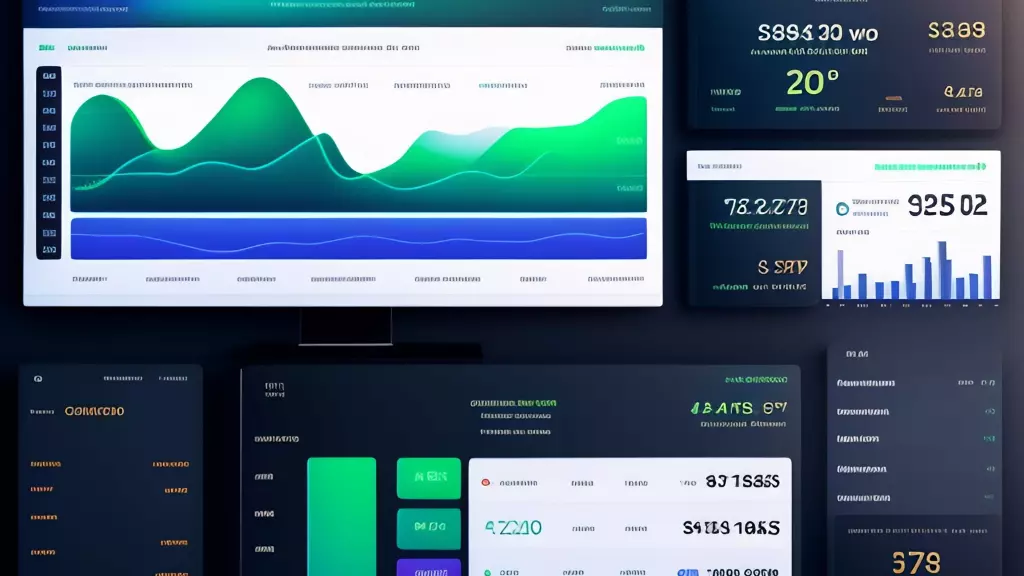
As the field continues to evolve, graphical analytics will become an indispensable tool for unlocking the hidden potential within data. Also read about the Treasury retail securities: a low-risk, smart choice for beginners.
Pingback: Grow your business with youtube ads and be a highlight of your niche - Forex Optcom